Data-driven Interior Plan Generation for Residential Buildings
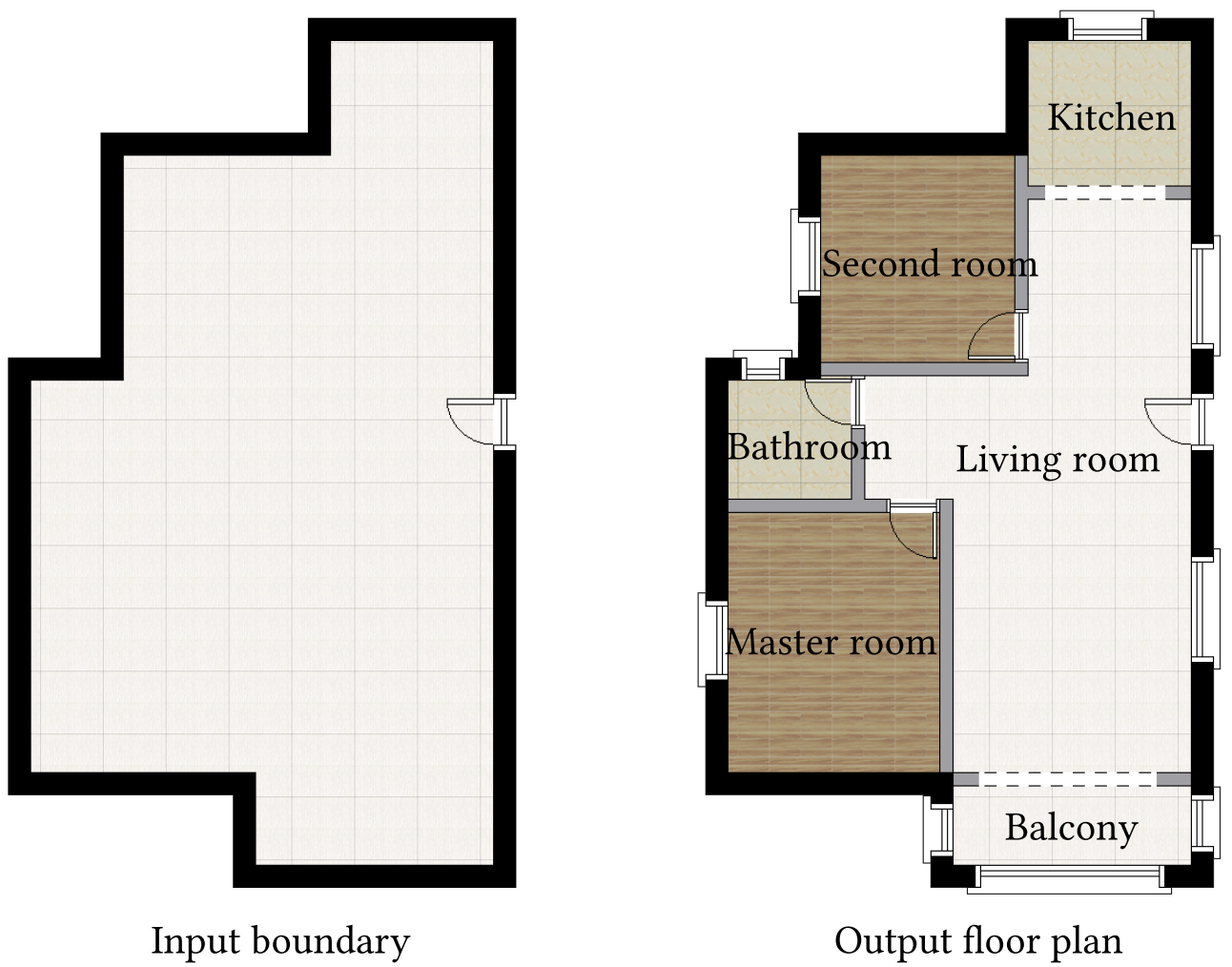
Figure 1: Floor plan for one residential building generated by our approach, given a boundary as input.
Abstract
We propose a novel data-driven technique for automatically and efficiently generating floor plans for residential buildings with given boundaries. Central to this method is a two-stage approach that imitates the human design process by locating rooms first and then walls while adapting to the input building boundary. Based on observations of the presence of the living room in almost all fl oor plans, our designed learning network begins with positioning a living room and continues by iteratively generating other rooms. Then, walls are first determined by an encoder-decoder network, and then they are refined to vector representations using dedicated rules. To effectively train our networks, we construct RPLAN - a manually collected large-scale densely annotated dataset of floor plans from real residential buildings. Intensive experiments, including formative user studies and comparisons, are conducted to illustrate the feasibility and efficacy of our proposed approach. By comparing the plausibility of different floor plans, we have observed that our method substantially outperforms existing methods, and in many cases our floor plans are comparable to human-created ones.
Downloads
Paper, Code, Data set: RPLAN, Slide, Video, Supp.
Bibtex
@article {Wu_DeepLayout_2019,
title = {Data-driven Interior Plan Generation for Residential Buildings},
author = {Wenming Wu and Xiao-Ming Fu and Rui Tang and Yuhan Wang and Yu-Hao Qi and Ligang Liu},
journal = {ACM Transactions on Graphics (SIGGRAPH Asia)},
volume = {38},
number = {6},
year = {2019},
}